AI has the potential to revolutionize the field of penetration testing by automating many repetitive, rote tasks like exploit development, vulnerability scanning, and report generation, thereby speeding up pen tests and making them more efficient.
However, AI is not yet advanced enough to fully replace the critical thinking and creativity required for human expertise, especially when it comes to testing custom web applications and proprietary systems.
Sample Network Pen Test Report
What should a penetration test report include? Download our sample report to learn.
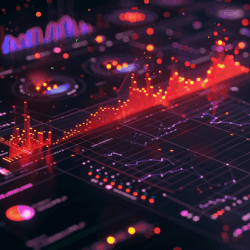
While AI offers significant advantages, there are risks associated with its integration, such as false positives, false negatives, scope creep, and accidental system crashes, necessitating skilled human oversight.
As a result, the roles of penetration testers may evolve to focus more on validating AI tool output, conducting adversary simulations, and formulating high-level strategies rather than executing technical tasks.
In a recent discussion, two seasoned offensive security professionals, Shubham Khichi and Nathaniel Shere, shared their perspectives on the future of AI in penetration testing, highlighting both the promises and challenges of this emerging technology.
Learn More: Why You Should Learn AI In Cybersecurity
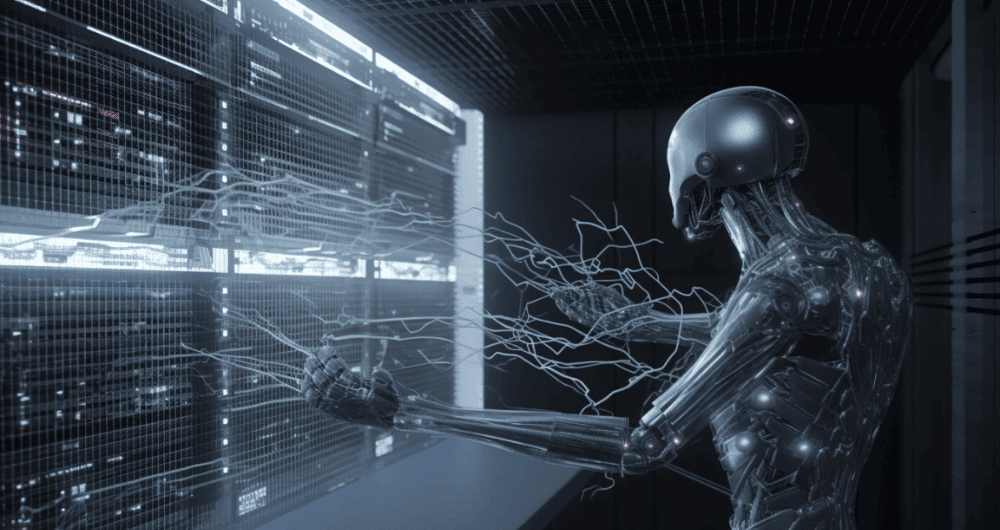
How Penetration Testing Has Evolved With AI
Shubham acknowledged the recent commercialization of AI, stating, “The commercialization does help us know a lot. We can query AI day and night and model it according to our testing needs.” He added, “It has a general intelligence if it is out there about the cybersecurity and testing tool, knowledge or scripting knowledge, it knows it after doing it is general intelligence.”
Nathaniel highlighted AI’s ability to automate repetitive tasks, saying:
“I know from a penetration testing perspective AI certainly helps automate a lot of the more rote things that pen testers have to do all the time.”
He provided an example:
“One of the easiest examples is quick exploit development. I haven’t seen every CVE even with ten years of experience. I haven’t seen every potential service.”
Learn More: How LLMs Are Being Exploited
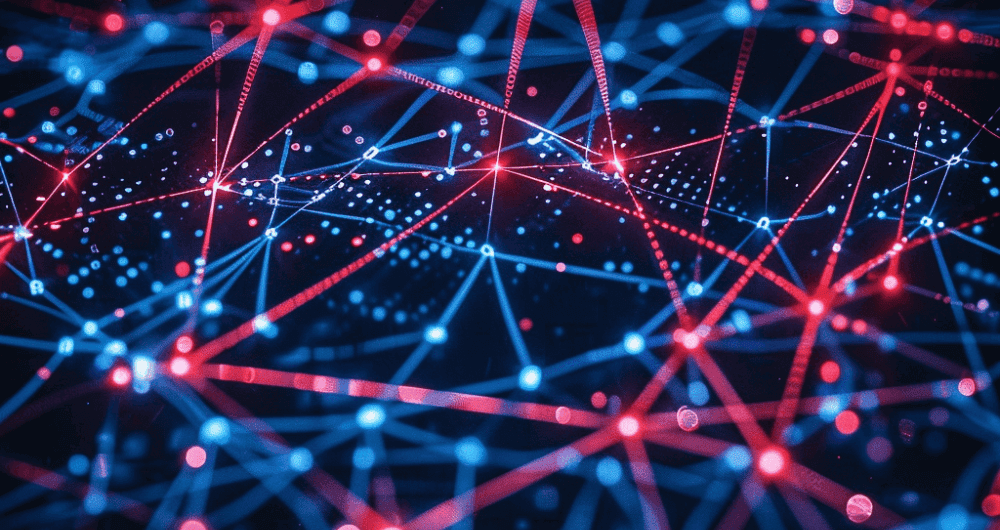
The Risks Of AI In Penetration Testing
While the potential benefits of integrating AI into penetration testing are evident, both Nathaniel and Shubham highlighted several critical risks that must be carefully considered and mitigated.
A fundamental concern raised by Nathaniel is the possibility of false positives and false negatives, which can undermine the reliability and effectiveness of AI-powered testing tools.
“You have your traditional false positives, false negatives that are going to be with any automated tool or anything like that. False positives, where it thinks it found something that’s not there or a false negative or something is there, but it didn’t find it,” he cautioned.
These inaccuracies can stem from various factors, including inadequate training data, model biases, or an inability to comprehend the nuanced context of a given testing scenario.
False positives can lead to wasted time and resources, while false negatives can result in critical vulnerabilities being overlooked, potentially exposing organizations to significant risks.
Shubham echoed similar concerns, stating, “The problem we are having currently with our platform is we don’t know when to stop it.” He elaborated, “When it becomes a cyberattack, that line is just a matter of human morale.”
While AI can automate certain aspects of penetration testing, human oversight and validation are essential to mitigate these risks.
Nathaniel touched on the issue of scope creep, emphasizing the need for human control:
“But the more I just let I take control, the more risk we have of it either crashing something, even though it shouldn’t have tested either an employee, a service, or a third party that it shouldn’t be accessing because those things aren’t in scope.”
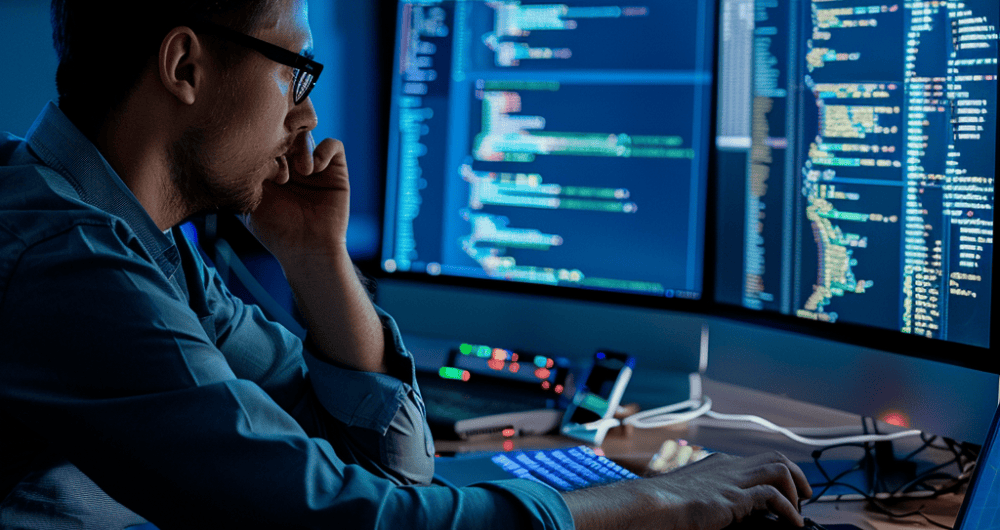
AI Replacing Human Penetration Testers
On the question of whether AI could fully replace human penetration testers, both experts expressed skepticism. Shubham said, “The web application part is the most difficult part to automate because technologies have developed so quickly and they’re so complicated. And there’s an entire industry of bug bounty hunters designed for this kind of work. You can’t replace that that field of cybersecurity.”
Nathaniel agreed, stating, “I’m very excited about AI taking away some of the more mundane, rote things like copying commands and pasting them. I could handle that, no problem. But in terms of losing my job over this. I don’t think so.”
Both experts acknowledged AI’s potential to streamline certain aspects of the penetration testing process but maintained that human expertise will continue to be indispensable.
Their perspectives highlight the nuanced relationship between AI and human intelligence, where the former can enhance the latter but is unlikely to replace it outright in fields that demand domain knowledge and creative problem-solving abilities.
Free Penetration Testing Policy
Skip the policy-writing hassle with our ready-to-use penetration testing policy template.
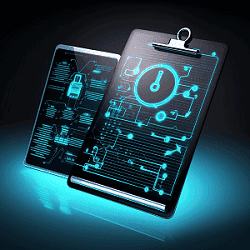
How AI Is Integrated Into Penetration Testing
As AI capabilities advance, offensive security professionals are exploring ways to integrate these technologies into their penetration testing practices.
Shubham highlighted the integration of AI in exploit development, report generation, and communication. “I do have a dog in the race with this question because the way we envision the way we use machine learning at this moment is you can’t use GPT-4 or 5 for this specific domain, you have to build your own even if it is from scratch or fine-tune using RAG. You have to do it. That is one way you can integrate it.”
Nathaniel also mentioned the use of AI in report generation and communication, saying, “I’ve seen tons of people talking about that. AI is taking over some report generation.”
The ability to automatically generate well-structured reports based on testing findings could significantly improve the efficiency of penetration testing engagements.
However, both experts acknowledged the need for human validation and oversight, as AI output may lack critical context or make unintended mistakes.
As Nathaniel noted:
“I have not seen anybody just using AI to automate communication with the client. I think that would be a little step one too far.”
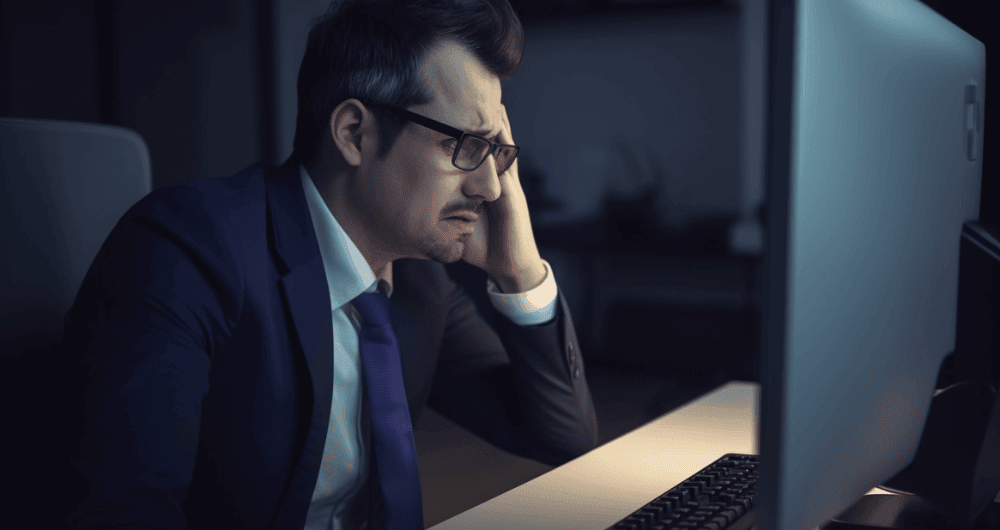
Challenges Of Deploying AI For Penetration Testing
Integrating AI into penetration testing practices is not without its challenges. These challenges span technical complexities, data availability, and the need to maintain a client-centric approach.
From a technical standpoint, Shubham emphasized the inherent difficulty of understanding and implementing machine learning techniques effectively.
“Understanding the entirety of machine learning is the biggest hurdle. This entire technology of machine learning, the amount of epochs needed, the amount of data sets needed. It’s very, very complicated,” he said.
Moreover, Shubham identified data scarcity as a critical challenge in the cybersecurity domain. “The biggest challenge is we don’t have large enough data sets to create a model on its own in cybersecurity,” he noted.
Unlike other fields with abundant data, the cybersecurity industry has a relatively short history, leading to limited availability of diverse and representative data for training AI models.
To overcome this hurdle, Shubham suggested the need for synthetic data generation techniques. “What if there was a way to, you know, generate that synthetic data and then populate your dataset to find, you know, training model? That’s the first hurdle and the biggest hurdle people will face,” he proposed.
While synthetic data can augment existing datasets, ensuring its accuracy and relevance to real-world scenarios remains a challenge.
Nathaniel, on the other hand, highlighted the importance of maintaining a client-centric approach when deploying AI for penetration testing.
“When I’m starting an engagement, I’m working with the client, understanding the scope, understanding potentially what their goals are with the test more than just, ‘Hey, can you find the vulnerabilities?’ or what kind of data is important to you?” he explained.
Penetration testing is not a one-size-fits-all exercise; it requires a deep understanding of the client’s business, priorities, and risk tolerance.
Failing to account for these nuances could result in an AI-powered solution that falls short of meeting the client’s specific needs or overlooks critical assets.
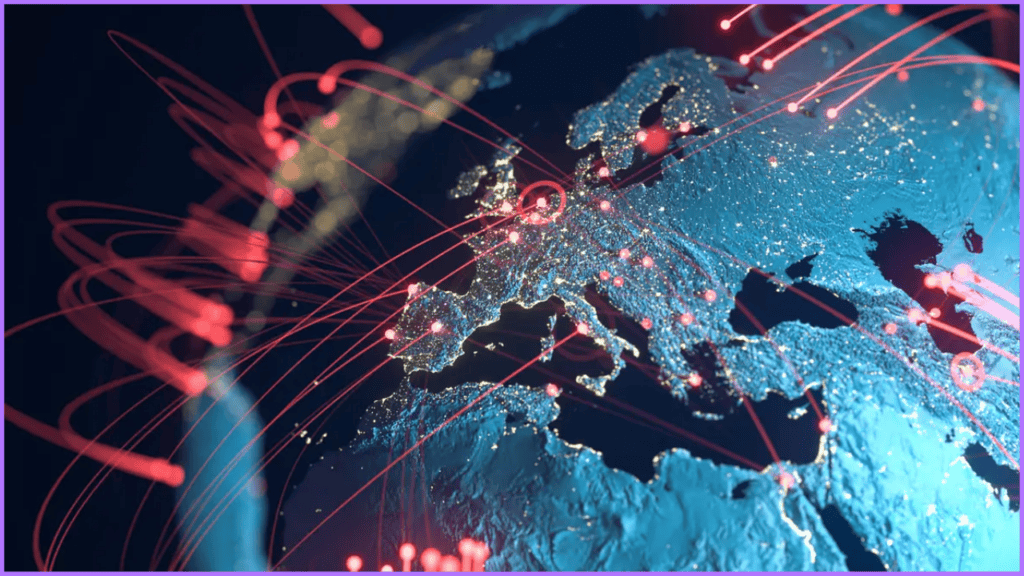
Future Trends Of AI In Penetration Testing
As AI capabilities continue to advance, both Nathaniel and Shubham envision transformative changes in the way penetration testing is conducted.
Traditional penetration testing engagements, often conducted annually or semi-annually due to resource constraints, leave organizations exposed to emerging threats during the interim periods.
Nathaniel sees AI-powered automation as a solution to this challenge, stating, “Being able to bring in a tool to get some more automation, to get almost real-time security testing on an ongoing basis, and then just validating the tool’s output maybe once, twice a year.”
Shubham, on the other hand, foresees a potential shift in hiring decisions as AI capabilities mature. “I think there will be an opportunity for CISOs where a doubt will be created, where they can actually compare. Should I even hire in this economy, a human versus a machine?” he posited.
However, both experts acknowledged that this transition would likely be gradual, with human oversight and validation remaining crucial in the foreseeable future.
As Shubham noted, “Obviously if a human is valuable, do it. Don’t skimp out on it, but then treat them nicely. Don’t lay them off after a year because we don’t have the budget. But if there’s a machine needed for your job, then be an advocate for it.”
$35/MO PER DEVICE
Enterprise Security Built For Small Business
Defy your attackers with Defiance XDR™, a fully managed security solution delivered in one affordable subscription plan.
Other Areas Of Security AI Is Being Integrated
While the conversation primarily revolved around the role of AI in penetration testing, both Nathaniel and Shubham acknowledged the technology’s potential to revolutionize other critical areas of cybersecurity, particularly in security operations centers (SOCs) and log analysis.
Nathaniel expressed excitement about the prospects of leveraging AI in SOC operations, stating, “So I’m sure it’s at least being started, but I’m very excited to see it on the SOC side, on the defense monitoring, alerting. I am very excited to see what I can do there.”
SOCs are tasked with the continuous monitoring of an organization’s networks, systems, and applications, often grappling with an overwhelming volume of security alerts and log data.
AI could play a pivotal role in streamlining this process by automating the correlation and prioritization of alerts, enabling faster response times and more efficient incident triage.
Shubham echoed the potential of AI in log analysis, acknowledging the challenges that human analysts face in processing and deriving insights from the massive volumes of log data generated by modern IT infrastructures.
“Along with penetration testing, ingestion of gigabytes of logs is a bigger problem for humans.”
However, as Shubham noted, a significant challenge in this domain is the scarcity of high-quality, log data for training AI models.
“The biggest challenge is we don’t have large enough data sets to create a model on its own in cybersecurity,” he stated, underscoring the need for innovative techniques like synthetic data generation to overcome this hurdle.
Conclusion
While AI is undoubtedly transforming the penetration testing landscape, these experts believe that human expertise will remain invaluable, particularly in areas such as web application testing and understanding business contexts.
However, AI’s potential to automate repetitive tasks, enhance reporting, and aid in log analysis suggests a future where AI complements and augments human capabilities rather than replacing them entirely.
Article by