The rise of large language models (LLMs) has brought about a new set of challenges and vulnerabilities. As organizations increasingly incorporate artificial intelligence (AI) into their products and services, it becomes important to understand the potential risks and how to mitigate them.
Sample Network Pen Test Report
What should a penetration test report include? Download our sample report to learn.
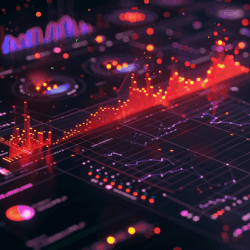
What Is An LLM?
LLMs or, large language models, are machine learning models that are trained and fine-tuned from scratch on a strata of data sets, usually stored in vector databases.
They are trained to answer questions by pulling data in a semantic search manner, hence the vector database use.
LLMs have revolutionized the way we interact with information, allowing users to receive concise and contextual answers by simply posing questions.
These models are trained on vast amounts of data, enabling them to provide relevant and accurate responses across a wide range of topics.
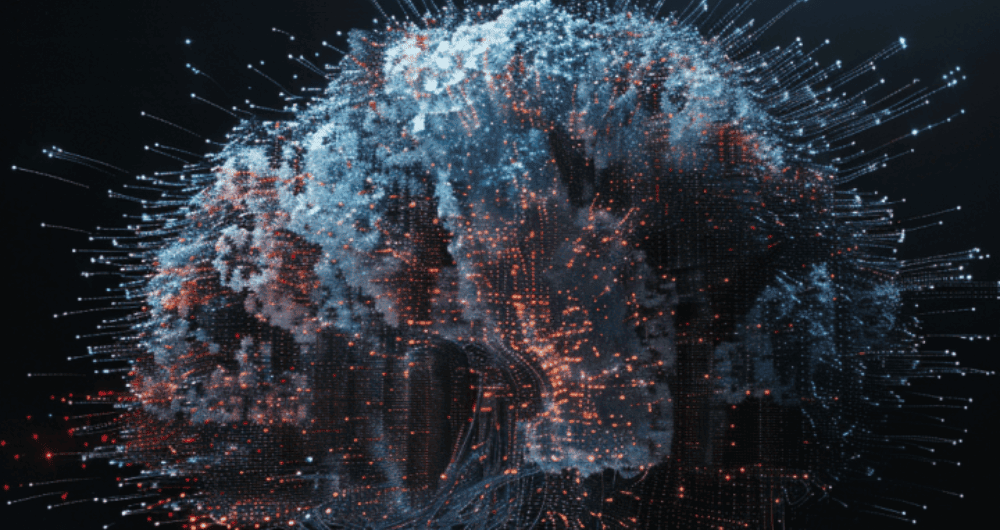
Common Vulnerabilities With LLMs
Despite their immense potential, LLMs are not immune to vulnerabilities. Shubham Khichi, founder and CEO of Nexus Infosec highlights some of the most prevalent issues:
The biggest vulnerability I’ve seen is actually it’s pretty human to say that it’s English. LLMs are trained on plain English, and the problem comes in if someone who articulates their words properly in a different tone and different manner, they can basically ask machines to do things for them.
One major vulnerability is the ability to bypass guard rails designed to prevent LLMs from providing offensive or harmful content which can be used by adversaries to develop malicious code or create social engineering campaigns.
Learn More: Why You Should Learn AI In Cybersecurity
Shubham elaborates:
There is a lot of adversary prompt engineering which good people in the offensive security community have come up with, where it can tell AI to get some information for them step by step. That is the biggest problem today, even though we have put guard rails, humans have figured a way out to get around it.
Additionally, data poisoning, where malicious actors introduce corrupted data into the training process, can significantly compromise the integrity and reliability of LLMs.
How LLMs Are Being Exploited
AI models have the potential to be exploited in various ways, including reconnaissance, reverse engineering, and even AI-on-AI attacks:
Many companies provide their open-source model for people to use, and usually, you can reverse engineer it to make it uncensored. Once you know the workings of that large language model, you can reverse engineer the Enterprise version as well.
Furthermore, Shubham warns about the possibility of AI models being used to attack and extract data from other AI models, stating:
If an AI understands another AI’s methodologies or languages or reverse engineers that non-stop 24/7, then there is a way to extract that data, get inside the company, and get all the user access.
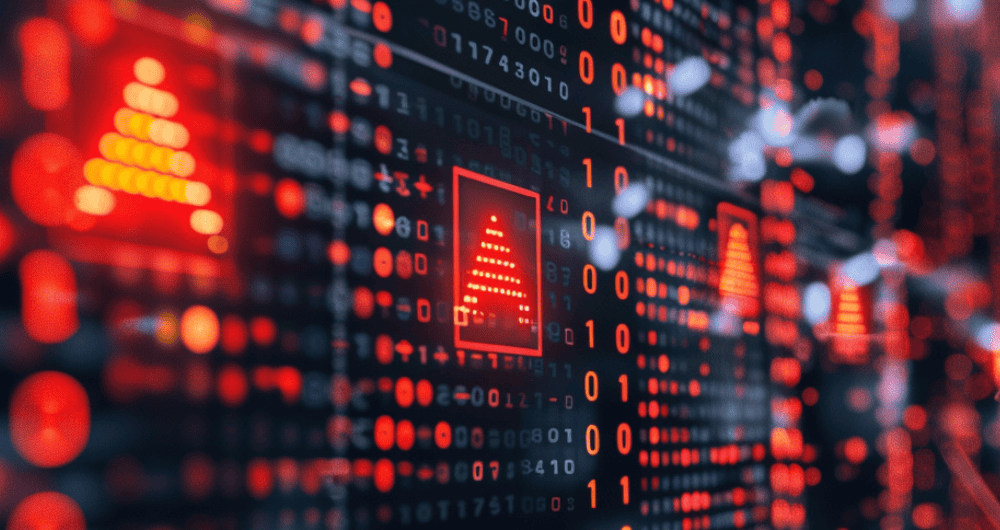
Defending Against LLM Exploits
Defending against LLM exploits is a daunting task, as the landscape is constantly evolving. Shubham emphasizes the importance of investing in security teams and resources:
Invest and invest tons of money and resources and manpower into your building your security team. Layoffs do happen, I understand that, but if you’re laying off your security team, you should pretty much say goodbye to your products and your stock prices.
In addition, he stresses the need for security professionals to evolve and adapt to the AI-driven landscape, transitioning from traditional penetration testing roles to become “adversary engineers” capable of defending against AI-based threats.
Learn More: Is AI The Future Of Penetration Testing?
Free Penetration Testing Policy
Skip the policy-writing hassle with our ready-to-use penetration testing policy template.
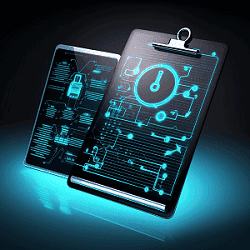
The Greatest Security Risks Of AI
According to Shubham, data is the primary security risk associated with AI:
You are no longer securing systems, you’re securing large language models and how they are stored, how they work, and you need to find out ways on identifying what could be possible in this model because every model is built different.
Understanding the unique challenges posed by AI models and identifying potential vulnerabilities specific to each model’s architecture and implementation is crucial for mitigating risks.
How Do We Make AI Secure?
Shubham acknowledges the difficulty in securing AI systems, stating, “I don’t know and it’s very shocking that I don’t know.” He attributes this uncertainty to two key factors:
- AI is a rapidly evolving field, and the threats are constantly changing, making it challenging to stay ahead of potential exploits.
- There is a lack of research and best practices specifically focused on securing organizations against AI-based threats.
However, Shubham suggests that the attacks may resemble traditional penetration testing methodologies, but with the added complexity of AI models attacking each other, further exacerbating the challenges.
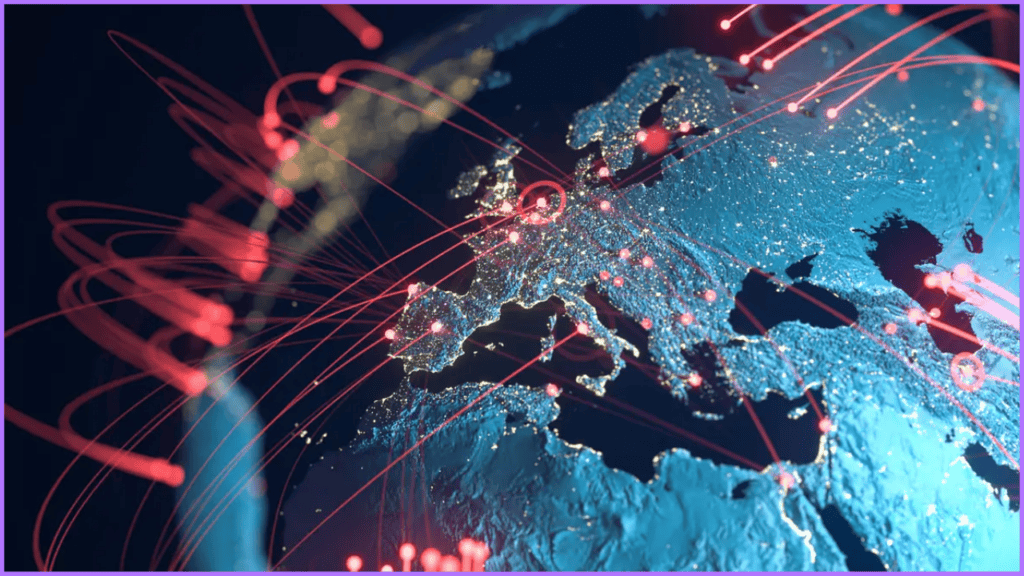
#1 Trend In AI Security
According to Shubham, prompt injection is currently the number one trend in AI security:
The biggest thing which I see is prompt injection, and that’s going to be for a long time unless there is extremely tight guard rails to ensure AI safety is not being breached no matter what happens.
Prompt injection involves crafting prompts in a specific manner to manipulate the AI model into providing information or performing actions it was not intended to.
As Shubham warns:
If you want to make a blog in the language which you want, you just name it, and it’s going to do it as long as you’ve given it pre-context and better prompts in the beginning.
Conclusion
As AI continues to be integrated into all aspects of our lives, we must remain vigilant and proactive in addressing the security challenges posed by these advanced technologies.
By fostering a deeper understanding of LLMs, their vulnerabilities, and potential exploitation techniques, we can better equip ourselves to defend against emerging threats.
The road to a secure AI future may be riddled with obstacles, but by investing in research, cultivating specialized expertise, and establishing robust security practices, we can pave the way for the responsible and ethical integration of AI.
Article by